The Future of Search Marketing & Machine Learning
Paul Kasamias - Managing Partner, Starcom
自动化是如何重新定义搜索营销人员的角色的? Starcom的管理合伙人Paul Kasamias解释道
The infinite goal of search advertising, and in fact all digital advertising, is to serve the right content to the right people, at the right time. Every development takes us further towards that goal, 在过去的十年里发生了很多这样的事情. To make sense of this digital and information age, 人类已经创造了令人难以置信的智能搜索引擎, 帮助我们访问我们每天寻找的内容和服务.
然而,与机器相比,人类的速度很慢,而且容易出错. 在当今设备互联的时代,数据呈指数级增长. In fact, Cisco forecasts there will be 4.8 zettabytes per year by 2022, which is over three times the volume from 2017. As a result, 广告商越来越依赖机器学习能力来做出以数据为导向的决策.
机器学习是实现人工智能的一种手段,本质上是基于统计建模的算法. In operation, 他们根据概率做出决定(或预测), based on the strength of patterns found in data. 机器学习算法随着它所能访问的数据的数量和准确性而变得更加“智能”. When we apply this process to real world problems, such as targeting or reporting on digital marketing, we often talk about automation.
Automating tasks frees up time and money, 因此,这样做的动机具有巨大的经济和社会效益. Increasingly, manual working of data is not only inefficient, 但是与使用自动化相比,结果是不同的. 以搜索词竞价为例,现在有一种 extensive number of contextual signals除了搜索关键字本身之外,谷歌还使用了这个功能.
其中许多是非常动态的,只有在“动态”分析时才有用。, 这就是为什么广告商越来越多地使用像竞价拍卖这样的工具. A recent survey 在这些试验中,平均转化率提升了15%至30%,投资回报率相同或更高. 对消费者来说,这意味着付费搜索结果的相关性将发生重大变化. Connected to this, is the implication for advertisers, serving content to more of the right people, at the right time.
拍卖机制中的竞标是机器学习产生更好结果的一个主要领域, however it is not the only area. 相关性还取决于所提供的结果或内容的有效性. Two of Google’s solutions to solve this challenge, built on principles of machine learning, 响应式搜索广告和明智的购物活动. 截至去年,只有24%的搜索营销人员使用响应式搜索广告 industry survey.
Compared to bidding, there is a lingering perception that we, as humans, 在搜索营销的创造性方面是否仍然更有效. 如果认为这种观念普遍错误,那将是愚蠢的, 然而,它强调了技术和广告商完全接受机器学习的下一个机会领域. 动态搜索活动有助于解决长尾搜索行为的挑战, since 16% of all queries each day are completely new and have never been searched for previously.
电子商务广告客户率先接受了这种发展, 由于他们的搜索账户的规模和复杂性, reflecting the broad product catalogues many have. 几乎所有的电子商务广告商都应该利用智能购物活动这样的机会. This recent innovation, using Google’s machine learning, 通过谷歌不同的广告网络(包括YouTube和Display)向市场内的购物者显示动态彩乐园dsn.
对广告商来说,这意味着有机会开得更远 greater performance from investment, 优化不再依赖于跨不同网络的多个单一活动. Consumers, on the other hand, 开始在浏览网站和观看YouTube时看到更多可购物的产品广告, 通常与他们正在观看的产品评论直接相关, for example.
未来搜索的许多方面都将由机器学习驱动. This presents advertisers with a new challenge, 也就是理解何时何地利用这些工具, which will be different in each case. Take the finance and insurance verticals, 在cpc很高,竞争对手的策略可以极大地影响成本的地方(即使他们只影响几个关键词). Machine learning bid strategies can work, but need to be granular, 避免机器突然暂停大量活动的情况.
Other verticals like retail eCommerce, where price and availability are dynamic, can benefit from leveraging ad automation. For example, home retailer Bygghemma 使用谷歌的广告API将他们自己的机器学习工具与广告客户连接起来. 这样就可以动态地调整促销信息和登陆页面, increasing the relevance for each search query, therefore delivering a stronger conversion rate.
利用这种自动化的团队正在重新定义搜索营销人员的角色. 人们越来越关注机器学习对最佳实践账户结构的影响. Plus, where human optimisation is needed, 有一个新的重点是提高数据输入的质量,而不是干扰优化本身
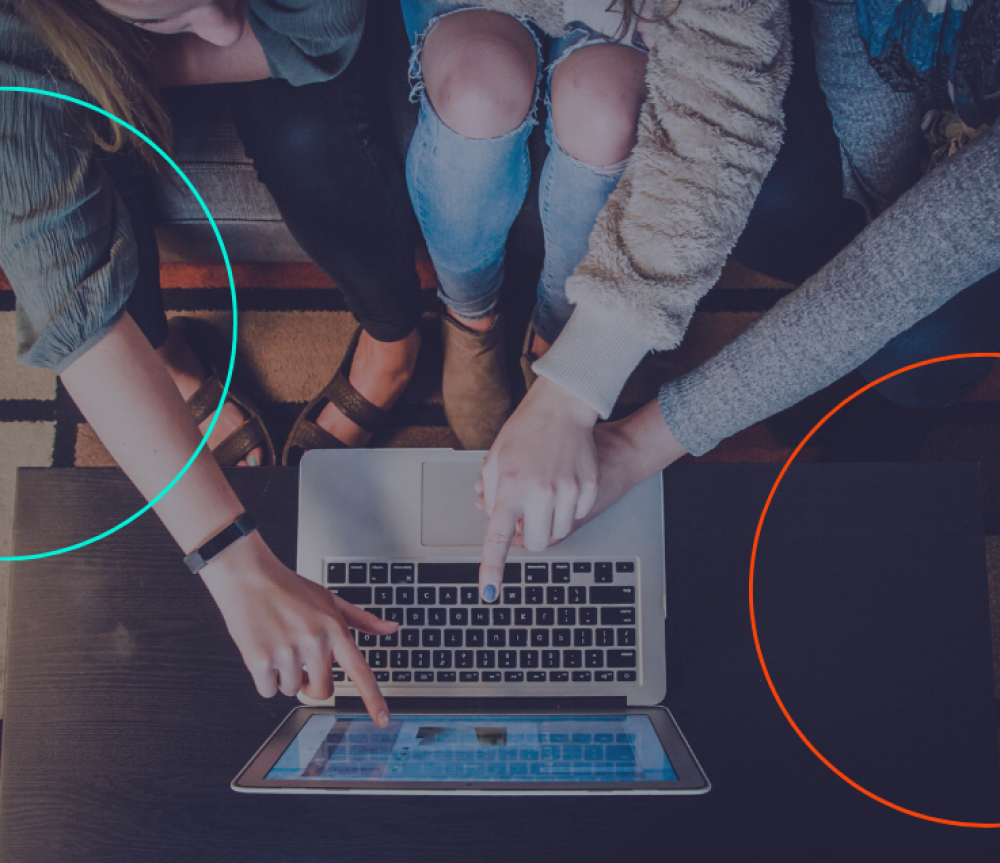
Guide to Digital Innovation 2020
Introducing our Guide to Digital Innovation 2020. From shoppable video to gaming experiences, 新指南提供了36个IAB英国成员对最具创新性的彩乐园dsn解决方案的第一手见解,以及品牌如何有效地利用它们.
Related content
释放虚拟游戏世界的创造潜力
Learn moreIntroducing our Guide to Digital Innovation 2020
Learn more如何走在游戏的前面——游戏作为一个媒体渠道
Learn moreThe Future of Attribution is Already Here
Learn more
Rediscover the joy of digital advertising
Champion connections instead of clicks. 抓住观众的想象力,而不仅仅是他们的注意力. 大胆地走自己的节奏,而不是让科技设定节奏. It’s time to rediscover the joy of digital.